Session 12: In-Orbit Demonstrations and Testbenches for On-Board Processing
Tracks
Day 4 - On-Board Processing Architectures
Thursday, June 17, 2021 |
5:15 PM - 6:35 PM |
Speaker
Eng. Fred Feresin
Institute of Research & Technology Saint Exupery
In-flight results of OPS-SAT images processing, by Artificial Neural Networks, embedded on FPGA
5:15 PM - 5:35 PMAbstract Submission
During the OBPDC-2020 conference held last year, we presented the publication “Onboard image processing using AI to reduce data transmission: example of OPS-SAT cloud segmentation”.
In this paper, we explained how we implemented three Artificial Neural Networks (ANNs) on OPS-SAT FPGA to perform cloud segmentation based on:
- A classical LeNet-5 architecture,
- A fully convolutional architecture,
- A hybrid convolutional / spiking architecture.
Cloud segmentation is a useful onboard service to filter unnecessary data and to preserve the limited storage and bandwidth of nanosats. This service is also compatible with OPS-SAT spatial resolution and the number of logic cells within its Cyclone V FPGA.
In the OBPDC-2020 paper, we detailed several challenges we had to tackle to achieve OPS-SAT implementation, specifically:
- Dataset engineering, which was made difficult by the fact that no actual OPS-SAT images were available at the time of ANN trainings,
- ANN architectures selection, which was almost completely driven by the execution target capability and required to come up with tiny designs,
- Hardware acceleration of the trained ANNs, using a VHDL based solution specifically developed to target OPS-SAT FPGA on Cyclone-V System on Chip.
In the continuity of the OBPDC-2020 paper, we propose for the OBDP-2021 conference to report the in-flight inferences of our ANNs on FPGA that is probably a world first. We will discuss the different parameters affecting the overall performance measured onboard OPS-SAT, while presenting, at least for one reference ANN, the impact of the different deployment steps on the inference metrics (in full precision on CPU, quantified on the validation board, and in-flight). Then we will propose relevant improvements.
We will especially analyze the generalization capability of the trained ANNs on real OPS-SAT images. Since these images display a wide variety of solar irradiance and geometry, we tested different kinds of pre-processing to handle this sensor behavior. We will therefore explain how we used the first images of OPS-SAT to create the new learning dataset.
We will also discuss the challenge of ANN quantization to perform inference on FPGA, with potential over or under flow depending on the selected arithmetic, applied not only to weights and biases but also on all the feature maps calculation. We will report the results of the solutions we tested, and we will propose further improvements.
The resulting pre and post processing time on HPS, and the inference time on FPGA, will be measured in-flight and compared to the on ground results.
Finally, some interesting information will be provided on the process that allowed, in coordination with ESOC team, uploading the full experiment onboard OPS-SAT: codes for the Hard Processor System (HPS) part, and bitstreams for the FPGA.
Depending on OPS-SAT availability before the conference, we could also present some improvements we intend to implement. In particular, we want to test an evolutionary algorithm as a complementary or challenging solution of the tiny Artificial Neural Networks.
In this paper, we explained how we implemented three Artificial Neural Networks (ANNs) on OPS-SAT FPGA to perform cloud segmentation based on:
- A classical LeNet-5 architecture,
- A fully convolutional architecture,
- A hybrid convolutional / spiking architecture.
Cloud segmentation is a useful onboard service to filter unnecessary data and to preserve the limited storage and bandwidth of nanosats. This service is also compatible with OPS-SAT spatial resolution and the number of logic cells within its Cyclone V FPGA.
In the OBPDC-2020 paper, we detailed several challenges we had to tackle to achieve OPS-SAT implementation, specifically:
- Dataset engineering, which was made difficult by the fact that no actual OPS-SAT images were available at the time of ANN trainings,
- ANN architectures selection, which was almost completely driven by the execution target capability and required to come up with tiny designs,
- Hardware acceleration of the trained ANNs, using a VHDL based solution specifically developed to target OPS-SAT FPGA on Cyclone-V System on Chip.
In the continuity of the OBPDC-2020 paper, we propose for the OBDP-2021 conference to report the in-flight inferences of our ANNs on FPGA that is probably a world first. We will discuss the different parameters affecting the overall performance measured onboard OPS-SAT, while presenting, at least for one reference ANN, the impact of the different deployment steps on the inference metrics (in full precision on CPU, quantified on the validation board, and in-flight). Then we will propose relevant improvements.
We will especially analyze the generalization capability of the trained ANNs on real OPS-SAT images. Since these images display a wide variety of solar irradiance and geometry, we tested different kinds of pre-processing to handle this sensor behavior. We will therefore explain how we used the first images of OPS-SAT to create the new learning dataset.
We will also discuss the challenge of ANN quantization to perform inference on FPGA, with potential over or under flow depending on the selected arithmetic, applied not only to weights and biases but also on all the feature maps calculation. We will report the results of the solutions we tested, and we will propose further improvements.
The resulting pre and post processing time on HPS, and the inference time on FPGA, will be measured in-flight and compared to the on ground results.
Finally, some interesting information will be provided on the process that allowed, in coordination with ESOC team, uploading the full experiment onboard OPS-SAT: codes for the Hard Processor System (HPS) part, and bitstreams for the FPGA.
Depending on OPS-SAT availability before the conference, we could also present some improvements we intend to implement. In particular, we want to test an evolutionary algorithm as a complementary or challenging solution of the tiny Artificial Neural Networks.
Mr Oskar Flordal
Unibap Ab
SpaceCloud Cloud Computing and In-Orbit Demonstration
5:35 PM - 5:55 PMAbstract Submission
Processing requirements are exponentially increasing to keep pace with the data volumes generated by increasingly “Big Data” sensors. These requirements are compounded when factoring in the data movements planned for future spacecraft constellation mesh networks, i.e. connected spacecraft infrastructures for on-orbit fleet management, autonomous sensor fusion, data storage, very low latency actionable information generation, and real-time communication.
Unibap AB and Troxel Aerospace Industries, Inc. have worked together to develop a heterogeneous radiation-tolerant onboard cloud computing hardware platform bringing terrestrial Internet-of-Things edge processing to space, e.g. Infrastructure as a Service, Big Data analytics and Artificial Intelligence. This platform is part of Unibap’s SpaceCloud ecosystem, which makes virtual servers and other resources dynamically available to customers. Leveraging its powerful heterogeneous hardware platform, the SpaceCloud framework has been developed with support by the European Space Agency (ESA) to enable rapid and flexible application development using containerized and isolated virtualization either for execution locally or on networked spacecraft. SpaceCloud allows exchange of information that is transparent between local or networked nodes to facilitate cooperation using a distributed mesh network communication architecture.
A node is any entity or subfunction, such as an application, or a vehicle, ground control station, sensor read-out module, cloud detection application, data base indexing etc., that is connected to the mesh network. Data exchanges may include intra-data processing between different software apps in a pipeline, or telemetry from on-orbit robotics-based nodes, commands from ground control nodes, science data fusion, etc. By exchanging pertinent data, nodes can act together to perform a task autonomously without requiring direct control or intervention by a central control node.
The focus of SpaceCloud is to enable commercial software to be reused onboard to decrease the overall cost and development time to deploy new capabilities on compatible space assets. As an example, SaraniaSat and Unibap have worked with L3Harris Geospatial to enable the geospatial intelligence software suite ENVI®/IDL® on SpaceCloud. A very low-latency onboard SpaceCloud application for detecting aircraft using ENVI®/IDL® and machine learning within 100 sq. km multispectral satellite imagery has been successfully developed and demonstrated.
The SpaceCloud framework executes on the iX5 and iX10 families of x86 radiation tolerant computer solutions featuring AMD multi-core CPU, GPU, Microsemi FPGA, and Intel Movidius Myriad X VPU accelerator and local high-speed solid-state storage. Radiation testing in the US has shown very promising results on both 28 nm and 14 nm processor nodes with high tolerance for single event latch-up (SEL) and total ionizing dose (TID).
To improve radiation tolerance, the SpaceCloud framework performs real-time software monitoring and FDIR through the SafetyChip feature working in tandem on the x86 software stack and an RTOS in a fault-tolerant configuration in FPGA. The concept has been developed with funding support from ESA. Radiation tolerance can be further increased by use of a single event upset (SEU) mitigating middleware that protects CPU and GPU processing.
This paper presents the SpaceCloud In-orbit Demonstration compute architecture and framework configuration as implemented in D-Orbit’s Wild Ride ION SCV mission due for launch in Q2 2021.
Unibap AB and Troxel Aerospace Industries, Inc. have worked together to develop a heterogeneous radiation-tolerant onboard cloud computing hardware platform bringing terrestrial Internet-of-Things edge processing to space, e.g. Infrastructure as a Service, Big Data analytics and Artificial Intelligence. This platform is part of Unibap’s SpaceCloud ecosystem, which makes virtual servers and other resources dynamically available to customers. Leveraging its powerful heterogeneous hardware platform, the SpaceCloud framework has been developed with support by the European Space Agency (ESA) to enable rapid and flexible application development using containerized and isolated virtualization either for execution locally or on networked spacecraft. SpaceCloud allows exchange of information that is transparent between local or networked nodes to facilitate cooperation using a distributed mesh network communication architecture.
A node is any entity or subfunction, such as an application, or a vehicle, ground control station, sensor read-out module, cloud detection application, data base indexing etc., that is connected to the mesh network. Data exchanges may include intra-data processing between different software apps in a pipeline, or telemetry from on-orbit robotics-based nodes, commands from ground control nodes, science data fusion, etc. By exchanging pertinent data, nodes can act together to perform a task autonomously without requiring direct control or intervention by a central control node.
The focus of SpaceCloud is to enable commercial software to be reused onboard to decrease the overall cost and development time to deploy new capabilities on compatible space assets. As an example, SaraniaSat and Unibap have worked with L3Harris Geospatial to enable the geospatial intelligence software suite ENVI®/IDL® on SpaceCloud. A very low-latency onboard SpaceCloud application for detecting aircraft using ENVI®/IDL® and machine learning within 100 sq. km multispectral satellite imagery has been successfully developed and demonstrated.
The SpaceCloud framework executes on the iX5 and iX10 families of x86 radiation tolerant computer solutions featuring AMD multi-core CPU, GPU, Microsemi FPGA, and Intel Movidius Myriad X VPU accelerator and local high-speed solid-state storage. Radiation testing in the US has shown very promising results on both 28 nm and 14 nm processor nodes with high tolerance for single event latch-up (SEL) and total ionizing dose (TID).
To improve radiation tolerance, the SpaceCloud framework performs real-time software monitoring and FDIR through the SafetyChip feature working in tandem on the x86 software stack and an RTOS in a fault-tolerant configuration in FPGA. The concept has been developed with funding support from ESA. Radiation tolerance can be further increased by use of a single event upset (SEU) mitigating middleware that protects CPU and GPU processing.
This paper presents the SpaceCloud In-orbit Demonstration compute architecture and framework configuration as implemented in D-Orbit’s Wild Ride ION SCV mission due for launch in Q2 2021.
Mrs. Samantha Wagner
Spire Global
On-the-ground testbed for AI/ML- assisted on-board-processing on nanosatellite platform: Brain in Space initiative
5:55 PM - 6:15 PMAbstract Submission
With support from the ESA’s Earth Observation Science for Society Programme and in cooperation with Φ-lab, Spire has created ‘Brain in Space’ - an on-ground testbed, accessible via a web-based interface , replicating Spire’s LEMUR 3U platform, the flagship of Spire’s global nanosatellite constellation of over 100 satellites. Brain in Space consists of much of the same systems that can be found on a flight version of our LEMUR 3U spacecraft including power and communications systems, processing payloads, onboard computer and other utilities. In addition to the standard LEMUR systems, the testbed includes the Google Coral, Jetson Nano and UP Myriad X. embedded edge AI/ML modules.
Spire is currently operating several computing platforms for in-situ data processing (including AI/ML applications) that are part of standard Spire SDR products (Zynq Ultrascale+, Jetson TX2i). Spire is planning to expand its computing capabilities in the near future, by launching more and new types of boards.
The AI/ML modules allow for scheduling, uploading and testing of AI/ML-powered applications to rapidly process space sensor data of various types and from different sources, i.e.: Automated Identification System (AIS), Automatic Dependent Surveillance - Broadcast (ADS-B), GNSS-RO or Space Environment Monitoring .
It also provides a test environment to trial different AI frameworks and algorithms for space applications.
Within this simulated environment, users are able to test how well the AI/ML computing platforms can support the development of advanced AI-enabled analytics and edge computing in space.
The use of easily accessible on-the-ground testing environment like Brain in Space enables acceleration of new services development, innovations within smart data processing and edge computing directly on-board of small satellites and, stress-testing new solutions ahead of the launch to space. It also creates an opportunity to pilot new, AI-ML - empowered ways of how nanosatellite constellations are operated and managed.
The “Brain in Space” was developed under a programme of, and funded by, the European Space Agency. The views expressed above are in no way to be taken to reflect the official opinion of the European Space Agency.
Mr Francisco Membibre
Deimos Space S.L.U.
PIL testing of the Optical On-board Image Processing Solution for EO-ALERT
6:15 PM - 6:35 PMAbstract Submission
EO-ALERT (http://eo-alert-h2020.eu/) is a European Commission H2020 project coordinated by DEIMOS Space, whose main objective is to obtain Earth Observation products with very low latency (<5 minutes) using global communications links. To carry it out, the satellite sensor data are processed on-board the flight segment to obtain the target products, which are alerts in our applications (ship detection and extreme weather). This on-board processing is achieved through efficient use of novel and advanced COTS technologies, including spin-in from other sectors, such as advanced Multi-Processor and FPGA (Zynq™ UltraScale+™ MPSoC from Xilinx®), multi-board reconfiguration, use of COTS elements for advanced processing or rapid-development (Linux OS, SDSoC™ from Xilinx® or OpenCV libraries) and high-speed interfaces.
In order to program this COTS device from a global perspective in high level languages, the platform concept has been used. It is based on the block design of the FPGA and the custom Linux created with the Petalinux tool. Once the platform is ready, the new tools for programming Xilinx® COTS allow using all board resources such as IP cores in the FPGA for hardware acceleration or the use of parallel processing frameworks like OpenMP to fork the data between the 4 available cores to increase the performance.
To process the sensor images and obtain the target products, artificial intelligence (AI) and machine learning (ML) algorithms developed in the project are implemented by migrating them to the target Hardware, taking into account the most efficient implementation on the system multi-core (4 A53 ARM® cores) or FPGA. The development includes the use of rapid prototyping tools to produce optimized hardware IP blocks and SW libraries.
In the implementation and verification phase, a PIL (Processor-In-the-Loop) testbench is created. The objective of the test bench is to have a multi-board breadboard representative of the final architecture, in which to validate the design and its performance. The execution times are measured in the PIL platform, obtaining at the present moment results within the requirements (<5 minutes) established in the project.
For that, the HW architecture allows a master-slave configuration, to be used for this on-board processing, thus reducing the total latency of the product generation or cover more area. On each board the processing takes advantage of the board’s resources (multi-core, FPGA) to obtain the processed products and alerts, which are centralised in the master processing board.
In addition, the results are highly significative due to the evaluation of the resulting processing system is performed experimentally using real Earth Observation data from a reference-image database, corresponding to the DEIMOS-2 VHR optical satellite and the multispectral SEVIRI instrument on the MSG satellite. Ground truth information from multiple sources is used in the verification phase. Furthermore, the performance of the initial algorithms is compared with those obtained in the Hardware, thus obtaining an evaluation of the effect of the Hardware implementation.
In order to program this COTS device from a global perspective in high level languages, the platform concept has been used. It is based on the block design of the FPGA and the custom Linux created with the Petalinux tool. Once the platform is ready, the new tools for programming Xilinx® COTS allow using all board resources such as IP cores in the FPGA for hardware acceleration or the use of parallel processing frameworks like OpenMP to fork the data between the 4 available cores to increase the performance.
To process the sensor images and obtain the target products, artificial intelligence (AI) and machine learning (ML) algorithms developed in the project are implemented by migrating them to the target Hardware, taking into account the most efficient implementation on the system multi-core (4 A53 ARM® cores) or FPGA. The development includes the use of rapid prototyping tools to produce optimized hardware IP blocks and SW libraries.
In the implementation and verification phase, a PIL (Processor-In-the-Loop) testbench is created. The objective of the test bench is to have a multi-board breadboard representative of the final architecture, in which to validate the design and its performance. The execution times are measured in the PIL platform, obtaining at the present moment results within the requirements (<5 minutes) established in the project.
For that, the HW architecture allows a master-slave configuration, to be used for this on-board processing, thus reducing the total latency of the product generation or cover more area. On each board the processing takes advantage of the board’s resources (multi-core, FPGA) to obtain the processed products and alerts, which are centralised in the master processing board.
In addition, the results are highly significative due to the evaluation of the resulting processing system is performed experimentally using real Earth Observation data from a reference-image database, corresponding to the DEIMOS-2 VHR optical satellite and the multispectral SEVIRI instrument on the MSG satellite. Ground truth information from multiple sources is used in the verification phase. Furthermore, the performance of the initial algorithms is compared with those obtained in the Hardware, thus obtaining an evaluation of the effect of the Hardware implementation.
Session Chairs
Thomas Firchau
Dlr
Daniel Lüdtke
German Aerospace Center (DLR)
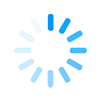