On-board cloud detection and selective spatial/spectral compression based on CCSDS 123.0-B-2 for hyperspectral missions
Tuesday, September 22, 2020 |
2:01 PM - 2:25 PM |
Speaker
Attendee25
Thales Alenia Space
On-board cloud detection and selective spatial/spectral compression based on CCSDS 123.0-B-2 for hyperspectral missions
Abstract Submission
Clouds are estimated to cover about 67% of Earth surface, and even after elimination of partially cloudy and cloud edge pixels, the total cloud cover remains close to 50%. Many applications which need to estimate Earth surface properties are useless in the presence of such opaque clouds, making half of the acquisitions unusable for Earth science applications.
Future hyperspectral satellite missions will provide numerous bands in VNIR and SWIR domain, with large swath and small spatial sampling distance. Therefore, the amount of data to be transmitted to ground is large, and image compression becomes mandatory.
CCSDS SLS-MHDC Working Group has established a recommended standard for a low-complexity data compression applied to multispectral and hyperspectral sensors. It provides an effective method of performing lossless or near-lossless compression, with a control of the error introduced, essential for scientific applications. The error can be band-dependent, however, the standard does not include a possibility for a selective compression in the spatial dimension.
Considering the significant presence of clouds, in particular for missions with continuous Earth acquisitions, the aim of this work, is to explore some possibilities to increase the hyperspectral compression performance on-board of satellite with a selective compression applied to clouds.
The first step of the compression scheme is the cloud detection. Cloud detection is widely used on-ground for cloud classification. Several methods are operational, such as the physical approach (or “threshold” approach) applied to Landsat and to Sentinel-2, or the approach based on Support Vector Machine (SVM) applied for example on a French commercial program. Some experiments have also been performed for satellite on-board detection with threshold approach.
For on-board compression purpose, only opaque clouds that hide the ground need to be detected. The threshold and the SVM approaches have been defined according to this need, and performance assessed on Landsat cloud data base. The SVM approach has been selected as it presents a high adaptability to evolutions and is simple to implement when based on well-chosen spectral bands. The SVM parameters are expected to be stable, and are defined on-ground, thanks to a training stage and to a consistent cloud data base. The SVM cloud detection is pixel-based, but it is followed by a simple filtering that requires few lines in order to reduce the false positive detection. The output of cloud detection is a spatial map, which identifies each hyperspectral pixel as a binary “cloud” or “ground”.
The principle of selective compression is to adapt the compression to the different classes. In the case of cloud compression, the objective is to apply a higher loss on the pixels detected as cloud compared to the ground pixels for an improved data rate reduction. Three different approaches have been studied, all based on the Multispectral and Hyperspectral image compression CCSDS standard. The first one applies a pre-quantization to the cloud pixels before getting into the compressor, which is still fully standard-compliant. The two other approaches occur inside the CCSDS compression - one by an adaptation of the prediction stage to the two classes of pixels - the other one by directly operating on the output of the prediction, thus incorporating non-standard features to support differentiated compression settings based on pixel class.
The performances of the three approaches have been assessed on hyperspectral AVIRIS images and on one simulated scene representative of the future Copernicus Hyperspectral Imaging Mission (CHIME). Implementation complexity has been assessed by Thales Alenia Space in Spain and University of Las Palmas de Gran Canaria, and is subject to another abstract submission.
This paper will give an overview of the cloud compression scheme, and will focus on the results obtained on the SVM cloud detection and on the cloud compression. Data rate reduction and impact on cloudy pixels after decompression will be presented together with some additional considerations to help for a selection of cloud compression scheme.
The study has been financed by ESA in the frame of CHIME phase A/B1 pre-developments.
The authors thank Manuel Sanchez from Thales Alenia Space Spain, Roberto Sarmiento, Yubal Barrios, Antonio Sánchez from University of Las Palmas de Gran Canaria for software development of CCSDS with evolutions and fruitful discussions, Mickael Bruno and Mathieu Albinet from CNES for CCSDS 123.0-B2 software used as reference.
Future hyperspectral satellite missions will provide numerous bands in VNIR and SWIR domain, with large swath and small spatial sampling distance. Therefore, the amount of data to be transmitted to ground is large, and image compression becomes mandatory.
CCSDS SLS-MHDC Working Group has established a recommended standard for a low-complexity data compression applied to multispectral and hyperspectral sensors. It provides an effective method of performing lossless or near-lossless compression, with a control of the error introduced, essential for scientific applications. The error can be band-dependent, however, the standard does not include a possibility for a selective compression in the spatial dimension.
Considering the significant presence of clouds, in particular for missions with continuous Earth acquisitions, the aim of this work, is to explore some possibilities to increase the hyperspectral compression performance on-board of satellite with a selective compression applied to clouds.
The first step of the compression scheme is the cloud detection. Cloud detection is widely used on-ground for cloud classification. Several methods are operational, such as the physical approach (or “threshold” approach) applied to Landsat and to Sentinel-2, or the approach based on Support Vector Machine (SVM) applied for example on a French commercial program. Some experiments have also been performed for satellite on-board detection with threshold approach.
For on-board compression purpose, only opaque clouds that hide the ground need to be detected. The threshold and the SVM approaches have been defined according to this need, and performance assessed on Landsat cloud data base. The SVM approach has been selected as it presents a high adaptability to evolutions and is simple to implement when based on well-chosen spectral bands. The SVM parameters are expected to be stable, and are defined on-ground, thanks to a training stage and to a consistent cloud data base. The SVM cloud detection is pixel-based, but it is followed by a simple filtering that requires few lines in order to reduce the false positive detection. The output of cloud detection is a spatial map, which identifies each hyperspectral pixel as a binary “cloud” or “ground”.
The principle of selective compression is to adapt the compression to the different classes. In the case of cloud compression, the objective is to apply a higher loss on the pixels detected as cloud compared to the ground pixels for an improved data rate reduction. Three different approaches have been studied, all based on the Multispectral and Hyperspectral image compression CCSDS standard. The first one applies a pre-quantization to the cloud pixels before getting into the compressor, which is still fully standard-compliant. The two other approaches occur inside the CCSDS compression - one by an adaptation of the prediction stage to the two classes of pixels - the other one by directly operating on the output of the prediction, thus incorporating non-standard features to support differentiated compression settings based on pixel class.
The performances of the three approaches have been assessed on hyperspectral AVIRIS images and on one simulated scene representative of the future Copernicus Hyperspectral Imaging Mission (CHIME). Implementation complexity has been assessed by Thales Alenia Space in Spain and University of Las Palmas de Gran Canaria, and is subject to another abstract submission.
This paper will give an overview of the cloud compression scheme, and will focus on the results obtained on the SVM cloud detection and on the cloud compression. Data rate reduction and impact on cloudy pixels after decompression will be presented together with some additional considerations to help for a selection of cloud compression scheme.
The study has been financed by ESA in the frame of CHIME phase A/B1 pre-developments.
The authors thank Manuel Sanchez from Thales Alenia Space Spain, Roberto Sarmiento, Yubal Barrios, Antonio Sánchez from University of Las Palmas de Gran Canaria for software development of CCSDS with evolutions and fruitful discussions, Mickael Bruno and Mathieu Albinet from CNES for CCSDS 123.0-B2 software used as reference.
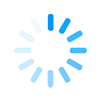